I’ve just spent Monday and Tuesday in New York for a workshop on ‘Museums + AI’. Funded by the AHRC and led by Oonagh Murphy and Elena Villaespesa, this was the second workshop in the year-long project.
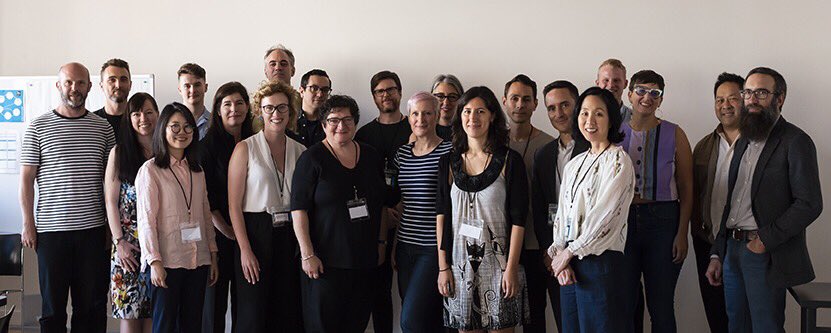
As there’s so much interest in artificial intelligence / machine learning / data science right now, I thought I’d revive the lost art of event blogging and share my notes. These notes are inevitably patchy, so keep an eye out for more formal reports from the team. I’ve used ‘museum’ throughout, as in the title of the event, but many of these issues are relevant to other collecting institutions (libraries, archives) and public venues. I’m writing this on the Amtrak to DC so I’ve been lazy about embedding links in text – sorry!
After a welcome from Pratt (check out their student blog https://museumsdigitalculture.prattsi.org/), Elena’s opening remarks introduced the two themes of the workshop: AI + visitor data and AI + Collections data. Questions about visitor data include whether museums have the necessary data governance and processes in place; whether current ethical codes and regulations are adequate for AI; and what skills staff might need to gain visitor insights with AI. Questions about collections data include how museums can minimise algorithmic biases when interpreting collections; whether the lack of diversity in both museum and AI staff would be reflected in the results; and the implications of museums engaging with big tech companies.
Achim Koh’s talk raised many questions I’ve had as we’ve thought about AI / machine learning in the library, including how staff traditionally invested with the authority to talk about collections (curators, cataloguers) would feel about machines taking on some of that work. I think we’ve broadly moved past that at the library if we can assume that we’d work within systems that can distinguish between ‘gold standard’ records created by trained staff and those created by software (with crowdsourced data somewhere inbetween, depending on the project).
John Stack and Jamie Unwin from the (UK) Science Museum shared some the challenges of using pre-built commercial models (AWS Rekognition and Comprehend) on museum collections – anything long and thin is marked as a 'weapon' – and demonstrated a nice tool for seeing 'what the machine saw' https://johnstack.github.io/what-the-machine-saw/. They don’t currently show machine-generated tags to users, but they’re used behind-the-scenes for discoverability. Do we need more transparency about how search results were generated – but will machine tags ever be completely safe to show people without vetting, even if confidence scores and software versions are included with the tags?
(If you’d like to see what all the tagging fuss is about, I have an older hands-on work sheet for trying text and images with machine classification software at https://www.openobjects.org.uk/2017/02/trying-computational-data-generation-and-entity-extraction/ )
Andrew Lih talked about image classification work with the Metropolitan Museum and Wikidata which picked up on the issue of questionable tags. Wikidata has a game-based workflow for tagging items, which in addition to tools for managing vandalism or miscreants allows them to trust the ‘crowd’ and make edits live immediately. Being able to sift incorrect from correct tags is vital – but this in turn raises questions of ‘round tripping’ – should a cultural institution ingest the corrections? (I noticed this issue coming up a few times because it’s something we’ve been thinking about as we work with a volunteer creating Wikidata that will later be editable by anyone.) Andrew said that the Met project put AI more firmly into the Wikimedia ecosystem, and that more is likely to come. He closed by demonstrating how the data created could put collections in the centre of networks of information http://w.wiki/6Bf Keep an eye out for the Wiki Art Depiction Explorer https://docs.google.com/presentation/d/1H87K5yjlNNivv44vHedk9xAWwyp9CF9-s0lojta5Us4/edit#slide=id.g34b27a5b18_0_435
Jeff Steward from Harvard Art Museums gave a thoughtful talk about how different image tagging and captioning tools (Google Vision, Imagga, Clarifai, Microsoft Cognitive Services) saw the collections, e.g. Imagga might talk about how fruit depicted in a painting tastes: sweet, juicy; how a bowl is used: breakfast, celebration. Microsoft tagger and caption tools have different views, don’t draw on each other.
Chris Alen Sula led a great session on ‘Ethical Considerations for AI’.
That evening, we went to an event at the Cooper Hewitt for more discussion of https://twitter.com/hashtag/MuseumsAI and the launch of their Interaction Lab https://www.cooperhewitt.org/interaction-lab/ Andrea Lipps and Harrison Pim’s talks reminded me of earlier discussion about holding cultural institutions to account for the decisions they make about AI, surveillance capitalism and more. Workshops like this (and the resulting frameworks) can provide the questions but senior staff must actually ask them, and pay attention to the answers. Karen Palmer’s talk got me thinking about what ‘democratising AI’ really means, and whether it’s possible to democratise something that relies on training data and access to computing power. Democratising knowledge about AI is a definite good, but should we also think about alternatives to AI that don’t involve classifications, and aren’t so closely linked to surveillance capitalism and ad tech?
The next day began with an inspiring talk from Effie Kapsalis on the Smithsonian Institution’s American Women’s History Initiative https://womenshistory.si.edu/ They’re thinking about machine learning and collections as data to develop ethical guidelines for AI and gender, analysing representations of women in multidisciplinary collections, enhancing data at scale and infusing the web with semantic data on historical women.
Shannon Darrough, MoMA, talked about a machine learning project with Google Arts and Culture to identify artworks in 30,000 installation photos, based on 70,000 collection images https://moma.org/calendar/exhibitions/history/identifying-art It was great at 2D works, not so much 3D, installation, moving image or performance art works. The project worked because they identified a clear problem that machine learning could solve. His talk led to discussion about sharing training models (i.e. once software is trained to specialise in particular subjects, others can re-use the ‘models’ that are created), and the alignment between tech companies’ goals (generally, shorter-term, self-contained) and museums’ (longer-term, feeding into core systems).
I have fewer notes from talks by Lawrence Swiader (American Battlefield Trust) with good advice on human-centred processes, Juhee Park (V&A) on frameworks for thinking about AI and museums, Matthew Cock (VocalEyes) on chat bots for venue accessibility information, and Carolyn Royston and Rachel Ginsberg (on the Cooper Hewitt’s Interaction Lab), but they added to the richness of the day. My talk was on ‘operationalising AI at a national library’, my slides are online https://www.slideshare.net/miaridge/operationalising-ai-at-a-national-library The final activity was on ‘managing AI’, a subject that’s become close to my heart.
Waves! And thank you for the summary, Mia!
cheers Mia, now i am really cross i missed it –
Thanks both! It's really good to know that it's useful. Sorry about your FOMO, Kevin!