A copy of my April 2023 position paper for the Collections as Data: State of the field and future directions summit held at the Internet Archive in Vancouver in April 2023. The full set of statements is available on Zenodo at Position Statements -> Collections as Data: State of the field and future directions. It'll be interesting to see how this post ages. I have a new favourite metaphor since I wrote this – the 'brilliant, hard-working — and occasionally hungover — [medical] intern'.
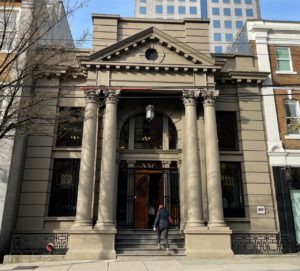
My favourite analogy for AI / machine learning-based tools[1] is that they’re like working with a child. They can spin a great story, but you wouldn’t bet your job on it being accurate. They can do tasks like sorting and labelling images, but as they absorb models of the world from the adults around them you’d want to check that they haven’t mistakenly learnt things like ‘nurses are women and doctors are men’.
Libraries and other GLAMs have been working with machine learning-based tools for a number of years, cumulatively gathering evidence for what works, what doesn’t, and what it might mean for our work. AI can scale up tasks like transcription, translation, classification, entity recognition and summarisation quickly – but it shouldn’t be used without supervision if the answer to the question ‘does it matter if the output is true?’ is ‘yes’.[2] Training a model and checking the results of an external model both require resources and expertise that may be scarce in GLAMs.
But the thing about toddlers is that they’re cute and fun to play with. By the start of 2023, ‘generative AI’ tools like the text-to-image tool DALL·E 2 and large language models (LLMs) like ChatGPT captured the public imagination. You’ve probably heard examples of people using LLMs as everything from an oracle (‘give me arguments for and against remodelling our kitchen’) to a tutor (‘explain this concept to me’) to a creative spark for getting started with writing code or a piece of text. If you don’t have an AI strategy already, you’re going to need one soon.
The other thing about toddlers is that they grow up fast. GLAMs have an opportunity to help influence the types of teenagers then adults they become – but we need to be proactive if we want AI that produces trustworthy results and doesn’t create further biases. Improving AI literacy within the GLAM sector is an important part of being able to make good choices about the technologies we give our money and attention to. (The same is also true for our societies as a whole, of course).
Since the 2017 summit, I’ve found myself thinking about ‘collections as data’ in two ways.[3] One is the digitised collections records (from metadata through to full page or object scans) that we share with researchers interested in studying particular topics, formats or methods; the other is the data that GLAMs themselves could generate about their collections to make them more discoverable and better connected to other collections. The development of specialist methods within computer vision and natural language processing has promise for both sorts of ‘collections as data’,[4] but we still have much to learn about the logistical, legal, cultural and training challenges in aligning the needs of researchers and GLAMs.
The buzz around AI and the hunger for more material to feed into models has introduced a third – collections as training data. Libraries hold vast repositories of historical and contemporary collections that reflect both the best thinking and the worst biases of the society that produced them. What is their role in responsibly and ethically stewarding those collections into training data (or not)?
As we learn more about the different ‘modes of interaction’ with AI-based tools, from the ‘text-grounded’, ‘knowledge-seeking’ and ‘creative’,[5] and collect examples of researchers and institutions using tools like large language models to create structured data from text,[6] we’re better able to understand and advocate for the role that AI might play in library work. Through collaborations within the Living with Machines project, I’ve seen how we could combine crowdsourcing and machine learning to clear copyright for orphan works at scale; improve metadata and full text searches with word vectors that help people match keywords to concepts rather than literal strings; disambiguate historical place names and turn symbols on maps into computational information.
Our challenge now is to work together with the Silicon Valley companies that shape so much of what AI ‘knows’ about the world, with the communities and individuals that created the collections we care for, and with the wider GLAM sector to ensure that we get the best AI tools possible.
[1] I’m going to use ‘AI’ as a shorthand for ‘AI and machine learning’ throughout, as machine learning models are the most practical applications of AI-type technologies at present. I’m excluding ‘artificial general intelligence’ for now.
[2] Tiulkanov, “Is It Safe to Use ChatGPT for Your Task?”
[3] Much of this thinking is informed by the Living with Machines project, a mere twinkle in the eye during the first summit. Launched in late 2018, the project aims to devise new methods, tools and software in data science and artificial intelligence that can be applied to historical resources. A key goal for the Library was to understand and develop some solutions for the practical, intellectual, logistical and copyright challenges in collaborative research with digitised collections at scale. As the project draws to an end five and a half years later, I’ve been reflecting on lessons learnt from our work with AI, and on the dramatic improvements in machine learning tools and methods since the project began.
[4] See for example Living with Machines work with data science and digital humanities methods documented at https://livingwithmachines.ac.uk/achievements
[5] Goldberg, “Reinforcement Learning for Language Models.” April 2023. https://gist.github.com/yoavg/6bff0fecd65950898eba1bb321cfbd81.
[6] For example, tools like Annif https://annif.org, and the work of librarian/developers like Matt Miller and genealogists.
Little, “AI Genealogy Use Cases, How-to Guides.” 2023. https://aigenealogyinsights.com/ai-genealogy-use-cases-how-to-guides/
Miller, “Using GPT on Library Collections.” March 30, 2023. https://thisismattmiller.com/post/using-gpt-on-library-collections/.